Have you started leveraging chatbots? If not, it is prime time to do it.
Today most websites and enterprises use chatbots for various purposes, and it offers them multiple benefits like cost advantage, saving on time, effort, and resources, 24/7 support, and much more. The chatbots that we have today can process and understand human languages and provide customized responses that enhance usersâ experience and engagement. How can a machine understand human languages and emotions? If you are wondering how then check this out.
This is possible by incorporating Natural language Processing (NLP) in chatbots. This blog will give you an in-depth overview of NLP and how it is changing usersâ experiences into a more realistic one.
What is NLP, and How did NLP come into existence?
Despite all our capabilities, processing vast amounts of data in a short period is a complex task for humans. Weâve realized that computers thrive in this field in ways that humans cannot. Computers do not use the same language as we do. They use binary and do not understand the context and nuances of human language and emotions. To bridge this gap, we need something extraordinary. This is where we need NLP.
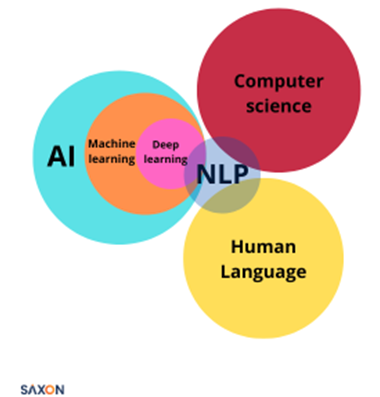
NLP is a combination of computer science, linguistics, and Artificial Intelligence that provides chatbots the ability to understand human languages and emotions. This makes the chatbots more human-like and serves their purpose better. You can use NLP in other virtual assistants like voice assistants or any domain-specific assistant and for other applications like machine translation, speech recognition, spam detection, information extraction, text mining, text summarization, etc.
History shows that NLP is not something new to us. It dates back to 1947, after world war II, when people realized the importance of language translation and hoped a machine could do the translation. With all the improvements over the years, now NLP is focused more on information extraction and generation from big data and uses the information to improve language processing.
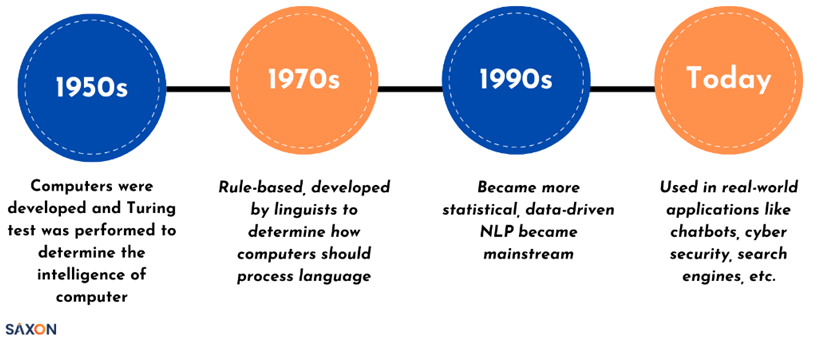
The Importance of NLP
Today businesses work with Big data. 90% of the data enterprises generate is unstructured and is in human language. Only a few organizations use all types of data to make data-driven decisions, and the rest do not follow the same practice because of the complexity involved in processing the unstructured data.
By leveraging NLP, businesses can extract useful information from unstructured data in no time. NLP can process even the vague elements in human languages that ML algorithms find hard to interpret. With the advent of deep learning, NLP can interpret all the elements effectively. This way, organizations can use their data effectively.
How does NLP work?
NLP is different from conventional machine learning algorithms in many aspects. The traditional ML algorithms can analyze only the structured data, whereas NLP can process unstructured data. Before processing the human language, it is necessary to understand how to deal with the
NLP empowers computers to understand natural language like humans do. Natural language processing uses artificial intelligence to process real-world inputs like voice commands or texts and understand the context. Algorithms read the texts, and the microphone captures the voice commands. Then converts the processed input to code that the computer can understand.
Natural language processing has two main processes
- Data Pre-processing
- Algorithm development
Data pre-processing is preparing and cleaning text data for machines to analyze. It converts data into a workable form and highlights features in the text that an algorithm can work on.
The following ways include data pre-processing.
- Tokenization is breaking down the sentences or text into tiny units.
- Stop word removal â Removes common words and retains the unique words.
- Stemming or lemmatization â Words are simplified to their root forms to process.
- Part-of-speech tagging â Words are tagged based on parts of speech like nouns, verbs, and adjectives.
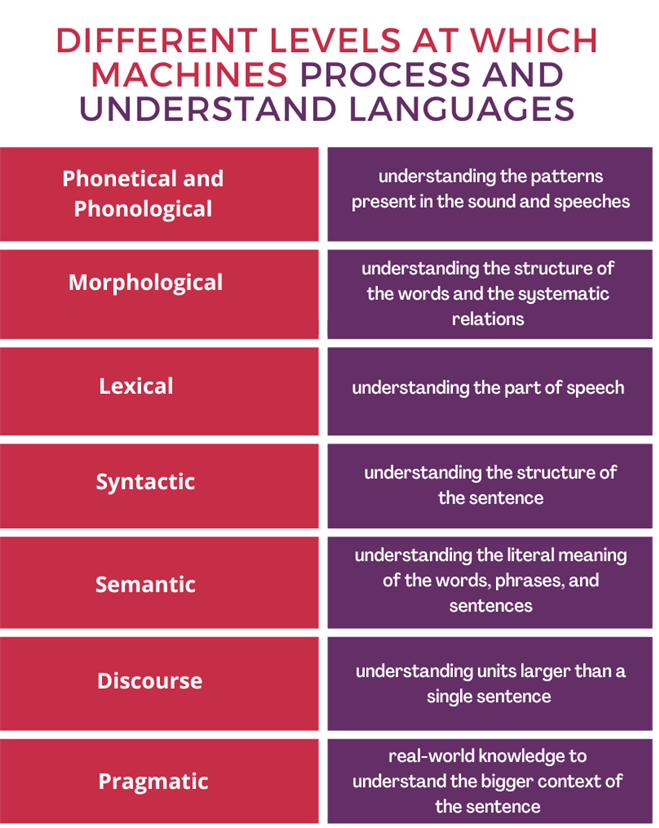
Once preprocessing is completed, it creates an algorithm to process the language. The commonly used algorithms are
- Rules-based system â This is an earlier practice that follows rules-based algorithms.
- Machine learning-based system â Machine learning algorithms employ statistical techniques. They learn to complete tasks using training data and refine their approaches as additional data is processed. Natural language processing algorithms refine their own rules through repeated processing and learning using a combination of machine learning algorithms, deep learning, and neural networks.
NLP = NLU + NLG
NLP has two sub-divisions, Natural Language Understanding (NLU) and Natural Language Generation (NLG).
Natural language Understanding can extract information like entity, relation, sentiment, and place. It involves two main techniques to process and understand text and speech. They are,
- Syntax analysis
- Semantic analysis
The Syntax refers to the parts of the sentences. NLP uses Syntax to analyze the meaning of the language formulated on grammatical rules. The syntax approach includes the following:
- Parsing is the grammatical analysis of the language.
Input â The bird sings
Noun | Bird |
Verb | Sings |
- Word segmentation differentiates the word from the empty white space.
- Sentence breaking sets sentence boundaries in lengthy texts.
- Morphological segmentation separates a word based on its syllables. This segmentation is useful in machine translation and speech recognition.
- Stemming breaks the words into their root words.
Semantics involves the usage and meaning of words. NLP uses algorithms to interpret the meaning and framework of sentences. Semantic techniques include:
- Word sense disambiguation â Interprets the meaning of a word based on context.
âThe tree bark is thick.â
The word bark has different meanings. The semantics analyzes the true meaning behind the word.
- Named entity recognition â This categorizes words into groups using semantics.
Example â âRichard Hiltonâs brother stayed at Hilton. The algorithm recognizes the two instances of âHiltonâ as two separate entities â one a hotel and one a person.
Speech recognition or speech to text â Converts live audio or recorded into a text document. Machine learning algorithms can extract features like intonation patterns that trigger phoneme sequences corresponding to specific word types. It will provide more accurate results than a transcription dictionary or language model.
Optical Character Recognition (OCR) â converts digital images or written text into machine readable form. With OCR in NLP, you can digitize your physical documents by scanning them.
Language identification â different languages are identified based on their rules and idiosyncrasies. It is essential to identify the language before the algorithm performs other actions. Deep neural networks aid in language translation.
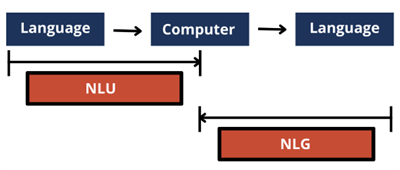
Natural language generation generates new text. NLG focuses on three main things. They are
- Data to text â unstructured data as input and generates text as output.
- Text to text â combines sources to give text output like summarization
- Dialogue â text is generated as conversations like chatbots
Example: An algorithm can autonomously write a summary of findings from a business intelligence platform, mapping certain words and phrases to data elements in the BI platform.
The Usage of NLP
You can use NLP across different industries and for multiple purposes. Most applications we use today have NLP. For example, letâs take Google search. When you type something on google search, It provides you with the word suggestions or completes your word. NLP does this. Proofreading and plagiarism checkers like Grammarly and Microsoft word use NLP.
Few use cases include,
- Sentiment analysis
- Automatic Language translation
- Intelligent virtual assistants
- Speech recognition
- Text summarization
- Document analysis
- Customer feedback analysis
- Customer service automation
- Academic research and analysis
- Analysis and categorization of medical records
- Stock forecasting and insights
- Talent recruitment in HR
- Automation of routine litigation tasks
Takeaway
Natural language processing plays a significant part in how we interact with technology today. As we progress towards a world of Robotic Process Automation (RPA), NLP will continue to boom. It has many real-world applications, and we have already started experiencing a world with NLP.
At SAXON, we build chatbots for all your needs. With an expert team, we can build chatbots for you in a week. Sounds perfect, right?
Get in touch with us to have your chatbot in just a week.